Smart Infrastructure and Data Analytics: Answering Key Questions
Reading time
Date posted
Written by Zhidong Li, one of the author of Data Analytics for Smart Infrastructure
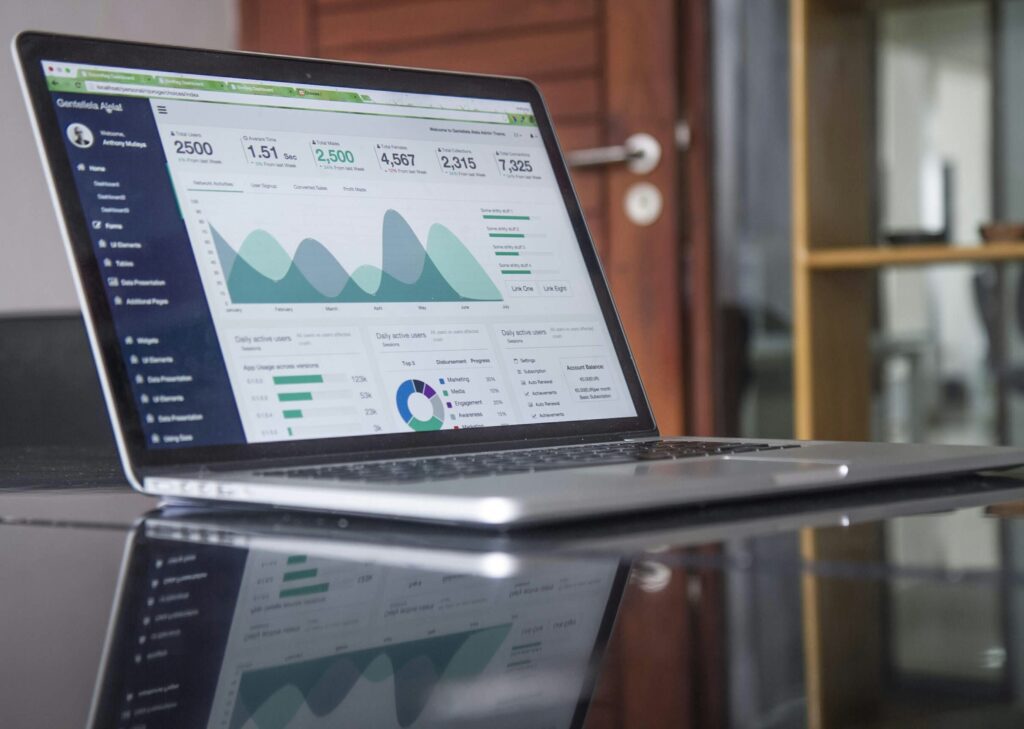
Photo by Carlos Muza on Unsplash
What inspired you to write Data Analytics for Smart Infrastructure, and what key message do you want readers to take away?
The book Data Analytics for Smart Infrastructure: Asset Management and Network Performance is inspired by the growing need for smart infrastructure in contemporary society. As infrastructure systems become increasingly complex and vast amounts of data are generated daily, leveraging advanced data analytics to improve efficiency, reliability, and sustainability has become crucial. The core goals of the book are to showcase the practical application of AI technologies in infrastructure, offering case studies that demonstrate how data-driven approaches can enhance management, such as performance analysis, anomaly detection, predictive analytics, and decision optimization. The book also aims to guide AI researchers to focus on the practical needs in infrastructure management, promoting the application of machine learning and data science techniques to real-world problems in this field. Additionally, the book presents and discusses potential challenges when integrating infrastructure data with AI systems, such as data quality, standardization, real-time processing, privacy, and security issues, and provides practical strategies to address these challenges. The central message of the book is that data analytics and artificial intelligence can significantly enhance the intelligence of infrastructure management, offering smarter, more efficient solutions to reduce maintenance costs, optimize resource allocation, and improve system reliability. Ultimately, the book aims to be a practical guide for professionals in the infrastructure field and inspire data scientists and AI researchers to apply their technologies to the development of smart infrastructure, driving societal progress.
Can you briefly explain how data analytics is transforming infrastructure asset management today?
Data analytics is transforming the way infrastructure asset management operates today, primarily in three key areas: Situation Awareness, Predictive Analytics, and Decision Support. Through data-driven methods, infrastructure managers can monitor asset conditions in real-time and identify anomalies. For example, in Structural Health Monitoring (SHM), sensor data combined with machine learning can automatically detect damage to infrastructure such as bridges and railways, reducing the cost of manual inspections and minimizing the risk of misjudgments. Predictive analytics shifts traditional maintenance from being schedule-based to condition-based, enabling predictive maintenance. For instance, pipeline burst predictions use machine learning to analyze historical data and identify potential failures in advance, optimizing repair schedules, and reducing maintenance costs and accident rates. Additionally, Decision Support powered by machine learning and big data helps managers optimize resource allocation and maintenance plans. For example, in railway systems, data-driven passenger flow predictions can optimize train scheduling, improving operational efficiency and service quality. Overall, data analytics is driving a shift in infrastructure management from passive maintenance to proactive prediction and optimization, enhancing asset utilization, reducing unexpected downtime, and increasing overall system reliability and sustainability.
Among the case studies in your book, which one do you find most compelling, and why?
One of the most captivating case studies in this book is the Structural Health Monitoring of the Sydney Harbour Bridge. Why is this case so special? The Sydney Harbour Bridge, an iconic Australian landmark with nearly a century of history, supports thousands of vehicles every day. However, time and pressure have caused aging and damage to its structure. The challenge is how to monitor the bridge’s health accurately without affecting traffic, and this is where data analytics shines. Researchers installed smart vibration sensors at multiple connection points on the bridge to continuously collect structural data. They then employed machine learning and Tensor Decomposition techniques, successfully identifying known cracks and, even more impressively, detecting potential structural hazards before they could lead to serious consequences. Traditional manual inspections are time-consuming, expensive, and prone to overlooking details, but with data analytics, non-destructive testing and precise predictions allow for a significant reduction in maintenance costs while ensuring long-term safety. This means the future of infrastructure maintenance will shift from reactive repairs to proactive forecasting. If you’re interested in how data is transforming the real world or want to see how AI protects our cities, this case will be eye-opening. It not only demonstrates the power of data science but also highlights the future of smart infrastructure—ushering in a safer, more efficient, and sustainable world.
How do you see AI and machine learning shaping the future of smart infrastructure?
Imagine a future where bridges can “self-diagnose,” detecting cracks before they form and alerting engineers for repairs; where city water pipes can “predict” leaks, identifying weak points before major disruptions occur; where subway and bus systems intelligently forecast passenger flow and adjust schedules automatically to prevent overcrowding. This isn’t science fiction—it’s the reality AI and machine learning are shaping today. By analyzing sensor data, historical trends, and environmental changes, AI enables urban infrastructure to shift from reactive maintenance to proactive prevention. Smart traffic systems optimize signals for smoother commutes, intelligent power grids adjust electricity distribution in real-time to minimize waste, and entire cities become safer, more efficient, and more sustainable. The infrastructure of the future will no longer be lifeless steel and concrete—it will think, learn, and evolve, adapting to the ever-changing needs of the city and making everyday life more seamless and convenient. Are you ready for the AI-powered smart cities of tomorrow?
If you’re a professional in the infrastructure industry looking to incorporate data analytics into your projects, now is the perfect time! Data-driven infrastructure management is changing the game, making asset management more efficient, maintenance more precise, and operations smarter. The key to successfully applying data analytics starts with building a high-quality data foundation—ensuring accuracy, completeness, and structure by leveraging sensors, historical maintenance records, and real-time monitoring systems to set the stage for insightful analysis. Equally important is selecting the right analytical methods for your business needs; for example, anomaly detection algorithms work well for identifying structural damage in bridges, while time-series analysis might be a better fit for predicting water pipe failures. Moreover, data analysis shouldn’t be a “black box”—it needs to offer clear, actionable insights. Using visualization tools to present results helps engineers and managers quickly understand and respond to findings. A smart approach is to start small, with a pilot project—apply AI monitoring to a single subway station or a few bridges, evaluate the impact, and then scale up from there. Beyond the technology, fostering a data-driven culture within your team is essential. Encourage a mindset of making decisions based on data, and collaborate closely with data scientists and AI experts. Combining technical innovation with industry expertise is the most powerful way to unlock the full value of data analytics and drive smarter, more sustainable infrastructure management.
If you could apply one innovation from your research to a global infrastructure project, what would it be?
If I had to choose one innovation from this book to apply to a global infrastructure project, it would be the intelligent water pipe failure prediction system, making water supply networks more efficient and sustainable worldwide. Traditional pipe maintenance follows a reactive approach—fixing leaks after they occur—but AI and data analytics can completely transform this process. By leveraging machine learning to analyze sensor data, historical maintenance records, and environmental factors, we can predict which pipes are most likely to fail and perform preventive maintenance before disasters strike, reducing water waste, lowering repair costs, and preventing major supply disruptions. If implemented globally, especially in drought-prone regions, this technology could improve the lives of millions, ensuring more reliable water access and promoting long-term sustainability. The cities of the future won’t just be smart—they’ll be self-healing!

Data Analytics for Smart Infrastructure
This book presents, for the first time, data analytics for smart infrastructures. The authors draw on over a decade’s experience working with industry and demonstrating the capabilities of data analytics for infrastructure and asset management.